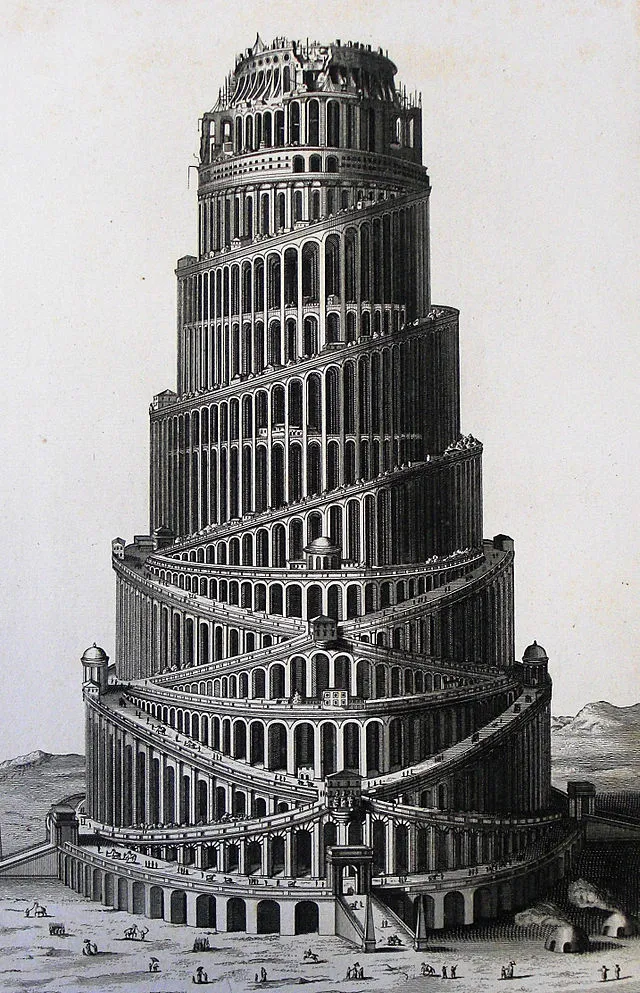
Large Language Models (ChatGPT) : Science & Stakes
ZOOM ACCESS IS FREE.
MEETING NUMBER IS 85718093402
PHYSICAL LOCALE IN MONTREAL: SHERBROOKE PAVILION (SH) LECTURE HALL SH-3620
DEDICATED TO THE MEMORY OF DANIEL C. DENNETT : 1942-2024
Summer School: June 3 – June 14, 2024
The Summer School is free for all on Zoom (with live webstreaming for the overflow on YouTube if needed). Registration is also free, and optional, but we recommend registering so you receive notices if there are any programme changes (and so we can know who and how many are attending). Questions and comments can only be aired if you provide your full name and institution. The video recordings for each of the 33 talks and 7 panels are accessible the day after they occur.
ZOOM ACCESS IS FREE.
MEETING NUMBER IS 85718093402
PHYSICAL LOCALE IN MONTREAL: SHERBROOKE PAVILION (SH) LECTURE HALL SH-3620
Speakers — Abstracts — Timetable
“Apart from what (little) OpenAI may be concealing from us, we all know (roughly) how ChatGPT works (its huge text database, its statistics, its vector representations, and their huge number of parameters, its next-word training, and so on). But none of us can say (hand on heart) that we are not surprised by what ChatGPT has proved to be able to do with these resources. This has even driven some of us to conclude that ChatGPT actually understands. It is not true that it understands. But it is also not true that we understand how it can do what it can do.” Language Writ Large
33 TALKS + 7 PANELS
Multimodal Vision-Language Learning Fri, June 14, 1:30pm-3pm EDT | AGRAWAL Aishwarya (U Montréal) computer-science GROUNDING |
Dimensionality and feature learning in Deep Learning and LLMs Mon, June 3, 1:30-3pm EDT | BELKIN Misha (UCSD) computer-science LEARNING |
Learning to reason is hard Tues, June 11, 9am-10:30am EDT | BENGIO Samy (Apple) computer-science LEARNING |
LLMs as Aids in Medical Diagnosis Mon June 10, 9:00am-10:30pm EDT | BZDOK Danilo (McGill) computer-science MEDICINE |
Stochastic Parrots or Emergent Reasoners: Can LLMs Understand? Mon, June 10, 1:30pm-3pm EDT | CHALMERS Dave (NYU) philosophy UNDERSTANDING |
How Do We Know What LLMs Can Do? Wed, June 12, 11am-12:30pm EDT | CHEUNG Jackie (McGill) computer-science UNDERSTANDING |
Language change Thurs, June 13, 11am-12:30pm EDT | DUBOSSARSKY Haim (Queen Mary Coll) computer science UNDERSTANDING |
“Will AI Achieve Consciousness? Wrong Question” Thurs, June 6, 11am-12:30pm EDT | IN MEMORIAM Daniel C. Dennett 1942-2024 |
We are (still!) not giving data enough crediti in computer vision Wed, June 12, 1:30pm-3pm EDT | EFROS Alyosha (Berkeley) computer-science GROUNDING |
The Physics of Communication Wed, June 5, 1:30pm-3pm EDT | FRISTON Karl (UCL) neuroscience PHYSICS |
The Place of Language Models in the Information-Theoretic Science of Language Mon, June 3, 9am-10:30am | FUTRELL Richard (UCI) linguistics LANGLEARNING |
Comparing how babies and AI learn language Tues, June 4, 11am-12:30pm EDT | GERVAIN Judit (U Padova) psychology LANGLEARNING |
Cognitive Science Tools for Understanding the Behavior of LLMs Fri, June 14, 3:30pm – 5pm EDT | GRIFFITHS Tom (Princeton) neuroscience/AI UNDERSTANDING |
**Special Daniel C. Dennett Memorial Talk** Thurs, June 6, 11am-12:30pm EDT | HUMPHREY Nick (Darwin Coll) psychology CONSCIOUSNESS |
Large Language Models and human linguistic cognition Wed, June 5, 11am-12:30pm EDT | KATZIR Roni (TAU) linguistics LANGLEARNING |
From Large Language Models to Cognitive Architectures Tues, June 11, 11am-12:30pm EDT | LEBIÈRE Christian (CMU) psychology COGNITION |
Missing Links: What still makes LLMs fall short of language understanding? Mon, June 10, 9am-10:30am EDT | LENCI Alessandro (Pisa) linguistics UNDERSTANDING |
Diverse Intelligence: the biology you need to make, and think about, AIs Tues, June 11, 1:30pm-3pm EDT | LEVIN Michael (Tufts) biology BIOLOGY |
What counts as understanding? Wed, June 12, 9am-10:30am EDT | LUPYAN Gary (Wisc) psychology UNDERSTANDING |
Semantic Grounding in Large Language Models Fri, June 14, 9am-10:30am EDT | LYRE Holger (Magdeburg) philosophy GROUNDING |
The Epistemology and Ethics of LLMs Thurs, June 6, 9am-10:30am EDT | MACLURE Jocelyn (McGill) philosophy ETHICS |
Using Language Models for Linguistics Fri, June 7, 9am-10:30am EDT | MAHOWALD Kyle (Texas) linguistics LANGLEARNING |
AI’s Challenge of Understanding the World Thurs, June 6, 3:30pm-5pm EDT | MITCHELL Melanie (SantaFe Inst) computer-science UNDERSTANDING |
Symbols and Grounding in LLMs Fri, June 14, 11am-12:30pm EDT | PAVLICK Ellie (Brown) computer-science GROUNDING |
What neural networks can teach us about how we learn language Tues, June 4, 9am-10:30am EDT | PORTELANCE Eva (McGill) cognitive-science LANGLEARNING |
Constraining networks biologically to explain grounding Mon, June 3, 11am-12:30pm EDT | PULVERMUELLER Friedemann (FU-Berlin) neuroscience GROUNDING |
Revisiting the Turing test in the age of large language models Thurs, June 13, 9am-10:30am EDT | RICHARDS Blake (McGill) neuroscience/AI UNDERSTANDING |
Emergent Behaviors & Foundation Models in AI Thurs, June 13, 1:30pm-3pm EDT | RISH Irina (MILA) computer-science LEARNING |
The Global Brain Argument Mon, June 3, 3:30pm-5pm EDT | SCHNEIDER Susan (Florida-Atlantic) philosophy CONSCIOUNESS |
Word Models to World Models: Natural to Probabilistic Language of Thought Thurs, June 6, 1:30pm-3pm EDT | TENENBAUM Josh (MIT) psychology UNDERSTANDING |
LLMs, POS and UG Tues, June 4, 1:30pm-3pm EDT | VALIAN Virginia (CUNY) Hunter psychology LANGLEARNING |
Computational Irreducibility, Minds, and Machine Learning Fri, June 7, 1:30pm-3pm EDT | WOLFRAM Stephen (Wolfram Research) computation MATHEMATICS |
Learning, Satisficing, and Decision Making Wed, June 5, 9am-10:30am EDT | YANG Charles (U Pennsylvania) linguistics LANGLEARNING |
Towards an AI Mathematician Fri, June 7, 11am-12:30pm EDT | YANG Kaiyu (Caltech) mathematics MATHEMATICS |
The Summer School is free for all on Zoom (with live webstreaming for the overflow on YouTube if needed). Registration is also free, and optional, but we recommend registering so you receive notices if there are any programme changes (and so we can know who and how many are attending). Questions and comments can only be aired if you provide your full name and institution. The video recordings for each of the 33 talks and 7 panels are accessible the day after they occur.
TIMETABLE
Speakers— Abstracts — Timetable
ZOOM ACCESS IS FREE.
MEETING NUMBER IS 85718093402
PHYSICAL LOCALE IN MONTREAL: SHERBROOKE PAVILION (SH) LECTURE HALL SH-3620
Speakers— Abstracts — Timetable
The Summer School is free for all on Zoom (with live webstreaming for the overflow on YouTube if needed). Registration is also free, and optional, but we recommend registering so you receive notices if there are any programme changes (and so we can know who and how many are attending). Questions and comments can only be aired if you provide your full name and institution. The video recordings for each of the 33 talks and 7 panels are accessible the day after they occur.
ZOOM ACCESS IS FREE.
MEETING NUMBER IS 85718093402
PHYSICAL LOCALE IN MONTREAL: SHERBROOKE PAVILION (SH) LECTURE HALL SH-3620
_________________________^top^
ABSTRACTS, BIOS, REFLINKS
_________________________
Multimodal Vision-Language Learning
Computer Science. University of Montreal, Mila
ISC Summer School on Large Language Models: Science and Stakes June 3-14, 2024
Fri, June 14, 1:30pm-3pm EDT
Abstract: Over the last decade, multimodal vision-language (VL) research has seen impressive progress. We can now automatically caption images in natural language, answer natural language questions about images, retrieve images using complex natural language queries and even generate images given natural language descriptions.Despite such tremendous progress, current VL research faces several challenges that limit the applicability of state-of-art VL systems. Even large VL systems based on multimodal large language models (LLMs) such as GPT-4V struggle with counting objects in images, identifying fine-grained differences between similar images, and lack sufficient visual grounding (i.e., make-up visual facts). In this talk, first I will present our work on building a parameter efficient multimodal LLM. Then, I will present our more recent work studying and tackling the following outstanding challenges in VL research: visio-linguistic compositional reasoning, robust automatic evaluation, and geo-diverse cultural understanding.
Aishwarya Agrawal is an Assistant Professor in the Department of Computer Science and Operations Research at University of Montreal. She is also a Canada CIFAR AI Chair and a core academic member of Mila — Quebec AI Institute. She also spends one day a week at Google DeepMind as a Research Scientist. Aishwarya’s research interests lie at the intersection of computer vision, deep learning and natural language processing, with the goal of developing artificial intelligence (AI) systems that can “see” (i.e. understand the contents of an image: who, what, where, doing what?) and “talk” (i.e. communicate the understanding to humans in free-form natural language).
Manas, O., Rodriguez, P., Ahmadi, S., Nematzadeh A., Goyal, Y., Agrawal A. MAPL: Parameter-Efficient Adaptation of Unimodal Pre-Trained Models for Vision-Language Few-Shot Prompting. In the European Chapter of the Association for Computational Linguistics (EACL), 2023
Zhang, L., Awal, R., Agrawal, A. Contrasting intra-modal and ranking cross-modal hard negatives to enhance visio-linguistic compositional understanding. In the IEEE Conference on Computer Vision and Pattern Recognition (CVPR), 2024
Manas, O., Krojer, B., Agrawal, A. Improving Automatic VQA Evaluation Using Large Language Models. In the 38th Annual AAAI Conference on Artificial Intelligence, 2024.
Ahmadi, S., Agrawal, A. An Examination of the Robustness of Reference-Free Image Captioning Evaluation Metrics. In the Findings of the Association for Computational Linguistics: EACL 2024
_________________________^top^
The puzzle of dimensionality and feature learning in modern Deep Learning and LLMs
Data Science, UCSD
ISC Summer School on Large Language Models: Science and Stakes, June 3-14, 2024
Mon, June 3, 1:30-3pm EDT
Abstract: Remarkable progress in AI has far surpassed expectations of just a few years ago and is rapidly changing science and society. Never before had a technology been deployed so widely and so quickly with so little understanding of its fundamentals. Yet our understanding of the fundamental principles of AI is lacking. I will argue that developing a mathematical theory of deep learning is necessary for a successful AI transition and, furthermore, that such a theory may well be within reach. I will discuss what such a theory might look like and some of its ingredients that we already have available. At their core, modern models, such as transformers, implement traditional statistical models — high order Markov chains. Nevertheless, it is not generally possible to estimate Markov models of that order given any possible amount of data. Therefore, these methods must implicitly exploit low-dimensional structures present in data. Furthermore, these structures must be reflected in high-dimensional internal parameter spaces of the models. Thus, to build fundamental understanding of modern AI, it is necessary to identify and analyze these latent low-dimensional structures. In this talk, I will discuss how deep neural networks of various architectures learn low-dimensional features and how the lessons of deep learning can be incorporated in non-backpropagation-based algorithms that we call Recursive Feature Machines.
Misha Belkin is a Professor at the Halicioglu Data Science Institute, University of California, San Diego, and an Amazon Scholar. His research interests are in theory and applications of machine learning and data analysis. His recent work has been concerned with understanding remarkable statistical phenomena observed in deep learning. One of the key recent findings is the “double descent” risk curve that extends the textbook U-shaped bias-variance trade-off curve beyond the point of interpolation. Mikhail Belkin is an ACM Fellow and a recipient of a NSF Career Award and a number of best paper and other awards. He is Editor-in-Chief of the SIAM Journal on Mathematics of Data Science.
Radhakrishnan, A., Beaglehole, D., Pandit, P., & Belkin, M. (2024). Mechanism for feature learning in neural networks and backpropagation-free machine learning models. Science, 383(6690), 1461-1467.
Belkin, M., Hsu, D., Ma, S., & Mandal, S. (2019). Reconciling modern machine-learning practice and the classical bias–variance trade-off. Proceedings of the National Academy of Sciences, 116(32), 15849-15854.
_________________________^top^
Learning to reason is hard
Senior Director, AI and Machine Learning Research, Apple
ISC Summer School on Large Language Models: Science and Stakes June 3-14, 2024
Tues, June 11, 9am-10:30am EDT
Abstract: Reasoning is the action of drawing conclusions efficiently by composing learned concepts. In this presentation I’ll give a few examples illustrating why it is hard to learn to reason with current machine learning approaches. I will describe a general framework (generalization of the unseen) that characterizes most reasoning problems and out-of-distribution generalization in general, and give insights about intrinsic biases of current models. I will then present the specific problem of length generalization and why some instances can be solved by models like Transformers and some cannot.
Samy Bengio is a senior director of machine learning research at Apple since 2021. His research interests span areas of machine learning such as deep architectures, representation learning, vision and language processing and more recently, reasoning. He co-wrote the well-known open-source Torch machine learning library.
Boix-Adsera, E., Saremi, O., Abbe, E., Bengio, S., Littwin, E., & Susskind, J. (2023). When can transformers reason with abstract symbols? arXiv preprint arXiv:2310.09753.ICLR 2024
Zhou,, E., Razin, N., Saremi, O., Susskind, J., … & Nakkiran, P. (2023). What algorithms can transformers learn? a study in length generalization. arXiv preprint arXiv:2310.16028. ICLR 2024
Abbe, E., Bengio, S., Lotfi, A., & Rizk, K. (2023, July). Generalization on the unseen, logic reasoning and degree curriculum. In International Conference on Machine Learning (pp. 31-60). PMLR. ICML 2023.
_________________________^top^
LLMs as Aids in Medical Diagnosis
Biomedical Engineering, McGill & MILA
ISC Summer School on Large Language Models: Science and Stakes, June 3-14, 2024
Mon, June 10, 11am-12:30pm EDT
Abstract: Despite considerable effort, we see diminishing returns in detecting people with autism using genome-wide assays or brain scans. In contrast, the clinical intuition of healthcare professionals, from longstanding first-hand experience, remains the best way to diagnose autism. In an alternative approach, we used deep learning to dissect and interpret the mind of the clinician. After pre-training on hundreds of millions of general sentences, we applied large language models (LLMs) to >4000 free-form health records from medical professionals to distinguish confirmed from suspected cases autism. With a mechanistic explanatory strategy, our extended LLM architecture could pin down the most salient single sentences in what drives clinical thinking towards correct diagnoses. It identified stereotyped repetitive behaviors, special interests, and perception-based behavior as the most autism-critical DSM-5 criteria. This challenges today’s focus on deficits in social interplay and suggests that long-trusted diagnostic criteria in gold standard instruments need to be revised.
Danilo Bzdok is an MD and computer scientist with a dual background in systems neuroscience and machine learning algorithms. His interdisciplinary research centers on the brain basis of human-defining types of thinking, with a special focus on the higher association cortex in health and disease. He uses population datasets (such as UK Biobank, HCP, CamCAN, ABCD) across levels of observation (brain structure and function, consequences from brain lesion, or common-variant genetics) with a broad toolkit of bioinformatic methods (machine-learning, high-dimensional statistics, and probabilistic Bayesian hierarchical modeling).
Bzdok, Danilo, et al. Data science opportunities of large language models for neuroscience and biomedicine. Neuron (2024).
Smallwood, J., Bernhardt, B. C., Leech, R., Bzdok, D., Jefferies, E., & Margulies, D. S. (2021). The default mode network in cognition: a topographical perspective. Nature Reviews Neuroscience, 22(8), 503-513.
Stanley, J., Rabot, E., Reddy, S., Belilovsky, E., Mottron, L., Bzdok, D (2024). Large language models deconstruct the clinical intuition behind diagnosing autism. Manuscript under review
_________________________^top^
Stochastic Parrots or Emergent Reasoners: Can Large Language Models Understand?
Center for Mind, Brain, and Consciousness, NYU
ISC Summer School on Large Language Models: Science and Stakes, June 3-14, 2024
Mon, June 10, 1:30pm-3pm EDT
Abstract: Some say large language models are stochastic parrots, or mere imitators who can’t understand. Others say that reasoning, understanding and other humanlike capacities may be emergent capacities of these models. I’ll give an analysis of these issues, analyzing arguments for each view and distinguishing different varieties of “understanding” that LLMs may or may not possess. I’ll also connect the issue of LLM understanding
David Chalmers is University Professor of Philosophy and Neural Science and co-director of the Center for Mind, Brain, and Consciousness at New York University. He is the author of The Conscious Mind (1996), Constructing The World (2010), and Reality+: Virtual Worlds and the Problems of Philosophy (2022). He is known for formulating the “hard problem” of consciousness, and (with Andy Clark) for the idea of the “extended mind,” according to which the tools we use can become parts of our minds.
Chalmers, D. J. (2023). Could a large language model be conscious?. arXiv preprint arXiv:2303.07103.
Chalmers, D.J. (2022) Reality+: Virtual worlds and the problems of philosophy. Penguin
Chalmers, D. J. (1995). Facing up to the problem of consciousness. Journal of Consciousness Studies, 2(3), 200-219.
Clark, A., & Chalmers, D. (1998). The extended mind. Analysis, 58(1), 7-19.
_________________________^top^
Benchmarking and Evaluation in NLP: How Do We Know What LLMs Can Do?
Computer Science, McGill University
ISC Summer School on Large Language Models: Science and Stakes, June 3-14, 2024
Wed, June 12, 11am-12:30pm EDT
Abstract: Conflicting claims about how large language models (LLMs) “can do X”, “have property Y”, or even “know Z” have been made in recent literature in natural language processing (NLP) and related fields, as well as in popular media. However, unclear and often inconsistent standards for how to infer these conclusions from experimental results bring the the validity of such claims into question. In this lecture, I focus on the crucial role that benchmarking and evaluation methodology in NLP plays in assessing LLMs’ capabilities. I review common practices in the evaluation of NLP systems, including types of evaluation metrics, assumptions regarding these evaluations, and contexts in which they are applied. I then present case studies showing how less than careful application of current practices may result in invalid claims about model capabilities. Finally, I present our current efforts to encourage more structured reflection during the process of benchmark design and creation by introducing a novel framework, Evidence-Centred Benchmark Design, inspired by work in educational assessment.
Jackie Chi Kit Cheung is associate professor, McGill University’s School of Computer Science, where he co-directs the Reasoning and Learning Lab. He is a Canada CIFAR AI Chair and an Associate Scientific Co-Director at the Mila Quebec AI Institute. His research focuses on topics in natural language generation such as automatic summarization, and on integrating diverse knowledge sources into NLP systems for pragmatic and common-sense reasoning. He also works on applications of NLP to domains such as education, health, and language revitalization. He is motivated in particular by how the structure of the world can be reflected in the structure of language processing systems.
Porada, I., Zou, X., & Cheung, J. C. K. (2024). A Controlled Reevaluation of Coreference Resolution Models. arXiv preprint arXiv:2404.00727.
Liu, Y. L., Cao, M., Blodgett, S. L., Cheung, J. C. K., Olteanu, A., & Trischler, A. (2023). Responsible AI Considerations in Text Summarization Research: A Review of Current Practices. arXiv preprint arXiv:2311.11103.
_________________________^top^
Will AI Achieve Consciousness? Wrong Question
IN MEMORIAM
(March 28, 1942 – April 19, 2024)
Dan Dennett, Professor Emeritus and Co-Director of the Center for Cognitive Studies, Tufts University, was a philosopher and cognitive scientist. A prominent advocate of evolutionary biology and computational models of the mind, Dennett is known for his theories on consciousness and free will, particularly the concept of consciousness as an emergent property of neural processes and evolution.
Levin, M., & Dennett, D. C. (2020). Cognition all the way down. Aeon Essays.
Dennett, D. C. (2020). The age of post-intelligent design. In: Gouveia, S. S. (Ed.). (2020). The age of artificial intelligence: An exploration. Vernon Press. 27.
Schwitzgebel, E., Schwitzgebel, D., & Strasser, A. (2023). Creating a large language model of a philosopher. Mind & Language 39(2) 237-259
_________________________^top^
Semantic Change
Queen Mary University, London
ISC Summer School on Large Language Models: Science and Stakes, June 3-14, 2024
Thurs, June 13, 11am-12:30pm EDT
Abstract: : Languages change constantly over time, influenced by social, technological, cultural, and political factors that alter our understanding of texts. New NLP/ML models can detect words that have changed their meaning. They help not only to understand old texts, but meaning changes across disciplines, the linguistic and social causes behind historical language change, changing views in political science and psychology, and changing styles in poetry and scientific writing. I will provide an overview of these computational models, describing changes in word categories and their implications for lexical evolution.
Haim Dubossarsky is Lecturer in the School of Electronic Engineering and Computer Science at Queen Mary University of London, and Affiliated Lecturer in the Language Technology Lab at the University of Cambridge. His research focuses on NLP and AI, with a special interest in the intersection of linguistics, cognition, and neuroscience, integrating advanced mathematical and computational methods across disciplines.
Cassotti, P., Periti, F., De Pascale, S., Dubossarsky, H., & Tahmasebi, N. (2024). Computational modeling of semantic change. In Proceedings of the 18th Conference of the European Chapter of the Association for Computational Linguistics: Tutorial Abstracts (pp. 1-8).
Periti, F., Cassotti, P., Dubossarsky, H., & Tahmasebi, N. (2024). Analyzing Semantic Change through Lexical Replacements. arXiv preprint arXiv:2404.18570.
Hahamy, A., Dubossarsky, H., & Behrens, T. E. (2023). The human brain reactivates context-specific past information at event boundaries of naturalistic experiences. Nature Neuroscience, 26(6), 1080-1089.
Dubossarsky, H., Tsvetkov, Y., Dyer, C., & Grossman, E. (2015). A bottom up approach to category mapping and meaning change. In NetWordS (pp. 66-70).
_________________________^top^
Lessons from Computer Vision: We are (still!) not giving Data enough credit
EECS, Berkeley
ISC Summer School on Large Language Models: Science and Stakes, June 3-14, 2024
Wed, June 12, 1:30pm-3pm EDT
Abstract: For most of Computer Vision’s existence, the focus has been solidly on algorithms and models, with data treated largely as an afterthought. Only recently did our discipline finally begin to appreciate the singularly crucial role played by data. In this talk, I will begin with some historical examples illustrating the importance of large visual data in both computer vision as well as human visual perception. I will then share some of our recent work demonstrating the power of very simple algorithms when used with the right data. Recent results in visual in-context learning, large vision models, and visual data attribution will be presented.
Alexei Efros is professor of electrical engineering and computer science at UC Berkeley. His research is on data-driven computer vision and its applications to computer graphics and computational photography using large amounts of unlabelled visual data to understand, model, and recreate the visual world. Other interests include human vision, visual data mining, robotics, and the applications of computer vision to the visual arts and the humanities. Visual data can also enhance the interaction capabilities of AI systems, potentially bridging the gap between visual perception and language understanding in robotics.
Bai, Y., Geng, X., Mangalam, K., Bar, A., Yuille, A., Darrell, T., Malik, J. and Efros, A.A. (2023). Sequential modeling enables scalable learning for large vision models. arXiv preprint arXiv:2312.00785.
Bar, A., Gandelsman, Y., Darrell, T., Globerson, A., & Efros, A. (2022). Visual prompting via image inpainting. Advances in Neural Information Processing Systems, 35, 25005-25017.
Pathak, D., Agrawal, P., Efros, A. A., & Darrell, T. (2017, July). Curiosity-driven exploration by self-supervised prediction. In International conference on machine learning (pp. 2778-2787). PMLR.
_________________________^top^
The Physics of Communication
Wellcome Centre for Human Neuroimaging, UCL London
ISC Summer School on Large Language Models: Science and Stakes, June 3-14, 2024
Wed, June 5, 1:30pm-3pm EDT
Abstract: The “free energy principle” provides an account of sentience in terms of active inference. Physics studies the properties that self-organising systems require to distinguish themselves from their lived world. Neurobiology studies functional brain architectures. Biological self-organization is an inevitable emergent property of any dynamical system. If a system can be differentiated from its external milieu, its internal and external states must be conditionally independent, inducing a “Markov blanket” separating internal and external states. This equips internal states with an information geometry providing probabilistic “beliefs” about external states. Bayesian belief updating can be demonstrated in the context of communication using simulations of birdsong. This “free energy” is optimized in Bayesian inference and machine learning (where it is known as an evidential lower bound). Internal states will appear to infer—and act on—their world to preserve their integrity.
Karl Friston, Director, Wellcome Centre for Human Neuroimaging, Institute of Neurology, UCL London is a theoretical neuroscientist and authority on brain imaging, invented statistical parametric mapping (SPM), voxel-based morphometry (VBM) and dynamic causal modelling (DCM). Mathematical contributions include variational Laplacian procedures and generalized filtering for hierarchical Bayesian model inversion. Friston currently works on models of functional integration in the human brain and the principles that underlie neuronal interactions. His main contribution to theoretical neurobiology is a free-energy principle for action and perception (active inference).
Pezzulo, G., Parr, T., Cisek, P., Clark, A., & Friston, K. (2024). Generating meaning: active inference and the scope and limits of passive AI. Trends in Cognitive Sciences, 28(2), 97-112.
Parr, T., Friston, K., & Pezzulo, G. (2023). Generative models for sequential dynamics in active inference. Cognitive Neurodynamics, 1-14.
Salvatori, T., Mali, A., Buckley, C. L., Lukasiewicz, T., Rao, R. P., Friston, K., & Ororbia, A. (2023). Brain-inspired computational intelligence via predictive coding. arXiv preprint arXiv:2308.07870.
Friston, K. J., Da Costa, L., Tschantz, A., Kiefer, A., Salvatori, T., Neacsu, V., … & Buckley, C. L. (2023). Supervised structure learning. arXiv preprint arXiv:2311.10300.
_________________________^top^
The Place of Language Models in the Information-Theoretic Science of Language
Language Science, UC Irvine
ISC Summer School on Large Language Models: Science and Stakes, June 3-14, 2024
Mon, June 3, 9am-10:30am
Abstract: Language models succeed in part because they share information-processing constraints with humans. These information-processing constraints do not have to do with the specific neural-network architecture nor any hardwired formal structure, but with the shared core task of language models and the brain: predicting upcoming input. I show that universals of language can be explained in terms of generic information-theoretic constraints, and that the same constraints explain language model performance when learning human-like versus non-human-like languages. I argue that this information-theoretic approach provides a deeper explanation for the nature of human language than purely symbolic approaches, and links the science of language with neuroscience and machine learning.
Richard Futrell, Associate Professor in the UC Irvine Department of Language Science, leads the Language Processing Group, studying language processing in humans and machines using information theory and Bayesian cognitive modeling. He also does research on NLP and AI interpretability.
Kallini, J., Papadimitriou, I., Futrell, R., Mahowald, K., & Potts, C. (2024). Mission: Impossible language models. arXiv preprint arXiv:2401.06416.
Futrell, R. & Hahn, M. (2024) Linguistic Structure from a Bottleneck on Sequential Information Processing. arXiv preprint arXiv:2405.12109.
Wilcox, E. G., Futrell, R., & Levy, R. (2023). Using computational models to test syntactic learnability. Linguistic Inquiry, 1-44.
_________________________^top^
Comparing how babies and AI learn language
Psychology, U Padua
ISC Summer School on Large Language Models: Science and Stakes, June 3-14, 2024
Tues, June 4, 11am-12:30pm EDT
Abstract: Judit Gervain will discuss the parallels and the differences between infant language acquisition and AI language learning, focusing on the early stages of language learning in infants. In particular, she will compare and contrast the type and amount of input infants and Large Language Models need to learn language, the learning trajectories, and the presence/absence of critical periods. She has used near-infrared spectroscopy (NIRS) as well as cross-linguistic behavioral studies to shed light on how prenatal linguistic exposure and early perceptual abilities influence language development. Her work has shown that infants discern patterns and grammatical structures from minimal input, a capability that AI systems strive to emulate.
Judit Gervain is professor of developmental and social psychology, University of Padua. Her research is on early speech perception and language acquisition in monolingual and bilingual infants. She using behavioral and brain imaging techniques. She has done pioneering work in newborn speech perception using near-infrared spectroscopy (NIRS), revealing the impact of prenatal experience on early perceptual abilities, and has been one of the first to document the beginnings of the acquisition of grammar in newborns and preverbal infants.
Mariani, B., Nicoletti, G., Barzon, G., Ortiz Barajas, M. C., Shukla, M., Guevara, R., … & Gervain, J. (2023). Prenatal experience with language shapes the brain. Science Advances, 9(47), eadj3524.
Nallet, C., Berent, I., Werker, J. F., & Gervain, J. (2023). The neonate brain’s sensitivity to repetition‐based structure: Specific to speech? Developmental Science, 26(6), e13408.
de la Cruz-Pavía, I., & Gervain, J. (2023). Six-month-old infants’ perception of structural regularities in speech. Cognition, 238, 105526.
______________________^top^
Cognitive Science Tools for Understanding the Behavior of Large Language Models
Computer Science, Princeton University
ISC Summer School on Large Language Models: Science and Stakes, June 3-14, 2024
FRI, June 14, 3:30PM-5pm EDT
Abstract: Large language models have been found to have surprising capabilities, even what have been called “sparks of artificial general intelligence.” However, understanding these models involves some significant challenges: their internal structure is extremely complicated, their training data is often opaque, and getting access to the underlying mechanisms is becoming increasingly difficult. As a consequence, researchers often have to resort to studying these systems based on their behavior. This situation is, of course, one that cognitive scientists are very familiar with — human brains are complicated systems trained on opaque data and typically difficult to study mechanistically. In this talk I will summarize some of the tools of cognitive science that are useful for understanding the behavior of large language models. Specifically, I will talk about how thinking about different levels of analysis (and Bayesian inference) can help us understand some behaviors that don’t seem particularly intelligent, how tasks like similarity judgment can be used to probe internal representations, how axiom violations can reveal interesting mechanisms, and how associations can reveal biases in systems that have bee trained to be unbiased.
Tom Griffiths is the Henry R. Luce Professor of Information Technology, Consciousness and Culture in the Departments of Psychology and Computer Science at Princeton University. His research explores connections between human and machine learning, using ideas from statistics and artificial intelligence to understand how people solve the challenging computational problems they encounter in everyday life. Tom completed his PhD in Psychology at Stanford University in 2005, and taught at Brown University and the University of California, Berkeley before moving to Princeton. He has received awards for his research from organizations ranging from the American Psychological Association to the National Academy of Sciences and is a co-author of the book Algorithms to Live By, introducing ideas from computer science and cognitive science to a general audience.
Yao, S., Yu, D., Zhao, J., Shafran, I., Griffiths, T., Cao, Y., & Narasimhan, K. (2024). Tree of thoughts: Deliberate problem solving with large language models. Advances in Neural Information Processing Systems, 36.
Hardy, M., Sucholutsky, I., Thompson, B., & Griffiths, T. (2023). Large language models meet cognitive science: Llms as tools, models, and participants. In Proceedings of the annual meeting of the cognitive science society (Vol. 45, No. 45).
_________________________^top^
Special Daniel C. Dennett Memorial Talk
Emeritus Professor of Psychology, LSE, Bye Fellow, Darwin College, Cambridge
ISC Summer School on Large Language Models: Science and Stakes, June 3-14, 2024
Thurs, June 6, 11am-12:30pm EDT
Abstract: Nicholas Humphrey will explore the concept of sentience as a crucial evolutionary development, discussing its role in human consciousness and social interactions. Sentience represents not just a biological but a complex psychological invention, crucial for personal identity and social fabric. He will also address Daniel Dennett’s Question “Will AI Achieve Consciousness? Wrong Question.”
Nicholas Humphrey, Emeritus Professor of Psychology at London School of Economics and Bye Fellow at Darwin College, Cambridge, did pioneering research on “blindsight,” the mind’s capacity to perceive without conscious visual awareness. His work on the evolution of human intelligence and consciousness emphasizes the social and psychological functions of sentience: how consciousness evolves, its role in human and animal life, and the sensory experiences that define our interaction with the world.
Humphrey, N., & Dennett, D. C. (1998). Speaking for our selves. Brainchildren: Essays on designing minds, ed. DC Dennett, 31-58. Analysis, 58(1), 7-19.
Humphrey, N. (2022). Sentience: The invention of consciousness. Oxford University Press.
_________________________^top^
Large Language Models and human linguistic cognition
Linguistics,Tel Aviv University
ISC Summer School on Large Language Models: Science and Stakes, June 3-14, 2024
Wed, June 5, 11am-12:30pm EDT
Abstract: Several recent publications in cognitive science have made the suggestion that Large Language Models (LLMs) have mastered human linguistic competence and that their doing so challenges arguments that linguists use to support their theories (in particular, the so-called argument from the poverty of the stimulus). Some of this work goes so far as to suggest that LLMs constitute better theories of human linguistic cognition than anything coming out of generative linguistics. Such reactions are misguided. The architectures behind current LLMs lack the distinction between competence and performance and between correctness and probability, two fundamental distinctions of human cognition. Moreover, these architectures fail to acquire key aspects of human linguistic knowledge and do nothing to weaken the argument from the poverty of the stimulus. Given that LLMs cannot reach or even adequately approximate human linguistic competence they of course cannot serve to explain this competence. These conclusions could have been (and in fact have been) predicted on the basis of discoveries in linguistics and broader cognitive science over half a century ago, but the exercise of revisiting these conclusions with current models is constructive: it points at ways in which insights from cognitive science might lead to artificial neural networks that learn better and are closer to human linguistic cognition.
Roni Katzir is an Associate Professor in the Department of Linguistics and a member of the Sagol School of Neuroscience at Tel Aviv University. His work uses mathematical and computational tools to study questions about linguistic cognition, such as how humans represent and learn their knowledge of language and how they use this knowledge to make inferences and contribute to discourse. Prof. Katzir received his BSc, in Mathematics, from Tel Aviv University and his PhD, in Linguistics, from the Massachusetts Institute of Technology. He heads Tel Aviv University’s Computational Linguistics Lab and its undergraduate program in Computational Linguistics and is a tutor in the Adi Lautman Interdisciplinary Program for Outstanding Students.
Lan, N., Geyer, M., Chemla, E., and Katzir, R. (2022). Minimum description length recurrent neural networks.Transactions of the Association for Computational Linguistics, 10:785–799.
Fox, D. and Katzir, R. (2024). Large language models and theoretical linguistics. To appear in Theoretical Linguistics.
Lan, N., Chemla, E., and Katzir, R. (2024). Large language models and the argument from the poverty of the stimulus. To appear in Linguistic Inquiry.
_________________________^top^
From Large Language Models to Cognitive Architectures
Psychology. Carnegie-Mellon University
ISC Summer School on Large Language Models: Science and Stakes, June 3-14, 2024
Tues, June 11, 11am-12:30pm EDT
Abstract: Large Language Models have displayed an uncanny ability to store information at scale and use it to answer a wide range of queries in robust and general ways. However, they are not truly generative in the sense that they depend on massive amounts of externally generated data for pre-training and lesser but still significant amounts of human feedback for fine-tuning. Conversely, cognitive architectures have been developed to reproduce and explain the structure and mechanisms of human cognition that give rise to robust behavior but have often struggled to scale up to real world challenges. We review a range of approaches to combine the two frameworks in ways that reflect their distinctive strengths. Moreover, we argue that, beyond their superficial differences, they share a surprising number of common assumptions about the nature of human-like intelligence that make a deep integration possible.
Christian Lebière is Research Faculty in the Psychology Department at Carnegie Mellon University, having received his Ph.D. from the CMU School of Computer Science. During his graduate career, he studied connectionist models and was the co-developer of the Cascade-Correlation neural network learning algorithm. He has participated in the development of the ACT-R cognitive architecture and most recently has been involved in defining the Common Model of Cognition, an effort to consolidate and formalize the scientific progress from the 50-year research program in cognitive architectures. His main research interests are cognitive architectures and their applications to artificial intelligence, human-computer interaction, intelligent agents, network science, cognitive robotics and human-machine teaming.
Laird, J. E., Lebiere, C., & Rosenbloom, P. S. (2017). A standard model of the mind: Toward a common computational framework across artificial intelligence, cognitive science, neuroscience, and robotics. AI Magazine, 38(4), 13-26.
Lebiere, C., Pirolli, P., Thomson, R., Paik, J., Rutledge-Taylor, M., Staszewski, J., & Anderson, J. R. (2013). A functional model of sensemaking in a neurocognitive architecture. Computational Intelligence and Neuroscience.
Lebiere, C., Gonzalez, C., & Warwick, W. (2010). Cognitive Architectures, Model Comparison, and Artificial General Intelligence. Journal of Artificial General Intelligence 2(1), 1-19.
Jilk, D. J., Lebiere, C., O’Reilly, R. C. and Anderson, J. R. (2008). SAL: an explicitly pluralistic cognitive architecture. Journal of Experimental & Theoretical Artificial Intelligence, 20(3), 197-218.
Anderson, J. R. & Lebiere, C. (2003). The Newell test for a theory of cognition. Behavioral & Brain Sciences 26, 587-637.
Anderson, J. R., & Lebiere, C. (1998). The Atomic Components of Thought. Mahwah, NJ: Lawrence Erlbaum Associates.
_________________________^top^
The Missing Links: What makes LLMs still fall short of language understanding?
Linguistics, U. Pisa
ISC Summer School on Large Language Models: Science and Stakes, June 3-14, 2024
Mon, June 10, 9am-10:30am EDT
ABSTRACT: The unprecedented success of LLMs in carrying out linguistic interactions disguises the fact that, on closer inspection, their knowledge of meaning and their inference abilities are still quite limited and different from human ones. They generate human-like texts, but still fall short of fully understanding them. I will refer to this as the “semantic gap” of LLMs. Some claim that this gap depends on the lack of grounding of text-only LLMs. I instead argue that the problem lies in the very type of representations these models acquire. They learn highly complex association spaces that correspond only partially to truly semantic and inferential ones. This prompts the need to investigate the missing links to bridge the gap between LLMs as sophisticated statistical engines and full-fledged semantic agents.
Alessandro Lenci is Professor of linguistics and director of the Computational Linguistics Laboratory (CoLing Lab), University of Pisa. His main research interests are computational linguistics, natural language processing, semantics and cognitive science.
Lenci A., & Sahlgren (2023). Distributional Semantics, Cambridge, Cambridge University Press.
Lenci, A. (2018). Distributional models of word meaning. Annual review of Linguistics, 4, 151-171.
Lenci, A. (2023). Understanding Natural Language Understanding Systems. A Critical Analysis. Sistemi Intelligenti, arXiv preprint arXiv:2303.04229.
Lenci, A., & Padó, S. (2022). Perspectives for natural language processing between AI, linguistics and cognitive science. Frontiers in Artificial Intelligence, 5, 1059998.
_________________________^top^
Diverse Intelligence: The biology you need to know in order to make, and think about, AI’s
Tufts University, Allen Discovery Center, and Harvard University, Wyss Institute
ISC Summer School on Large Language Models: Science and Stakes, June 3-14, 2024
Tues, June 11, 1:30pm-3pm EDT
Abstract: Human intelligence and that of today’s AI’s are just two points within a huge space of possible diverse intelligences. It is critical, for the development of both engineering advances and essential ethical frameworks, to improve our innately limited ability to recognize minds in unconventional embodiments. I will describe our work using the collective intelligence of body cells solving problems in anatomical and physiological spaces, to map out a framework for communicating with and understanding beings across the spectrum of cognition. By addressing fundamental philosophical questions through the lens of developmental biology and synthetic bioengineering, today’s AI debate can be greatly enriched.
Michael Levin is the director of the Allen Discovery Center at Tufts University. His lab works at the intersection of developmental biology, artificial life, bioengineering, synthetic morphology, and cognitive science. Seeking general principles of life-as-it-can-be, we use a wide range of natural animal models and also create novel synthetic and chimeric life forms. Our goal is to develop generative conceptual frameworks that help us detect, understand, predict, and communicate with truly diverse intelligences, including cells, tissues, organs, synthetic living constructs, robots, and software-based AIs. Our applications range from biomedical interventions to new ethical roadmaps.
Rouleau, N., and Levin, M. (2023), The Multiple Realizability of Sentience in Living Systems and Beyond, eNeuro, 10(11), doi:10.1523/eneuro.0375-23.2023
Clawson, W. P., and Levin, M. (2023), Endless forms most beautiful 2.0: teleonomy and the bioengineering of chimaeric and synthetic organisms, Biological Journal of the Linnean Society, 139(4): 457-486
Bongard, J., and Levin, M. (2023), There’s Plenty of Room Right Here: Biological Systems as Evolved, Overloaded, Multi-Scale Machines, Biomimetics, 8(1): 110
Fields, C., and Levin, M. (2022), Competency in Navigating Arbitrary Spaces as an Invariant for Analyzing Cognition in Diverse Embodiments, Entropy, 24(6): 819
Levin, M. (2022), Technological Approach to Mind Everywhere: an experimentally-grounded framework for understanding diverse bodies and minds, Frontiers in Systems Neuroscience, 16: 768201
_________________________^top^
What counts as understanding?
University of Wisconsin-Madison
ISC Summer School on Large Language Models: Science and Stakes, June 3-14, 2024
Wed, June 12, 9am-10:30am EDT
ABSTRACT: The question of what it means to understand has taken on added urgency with the recent leaps in capabilities of generative AI such as large language models (LLMs). Can we really tell from observing the behavior of LLMs whether underlying the behavior is some notion of understanding? What kinds of successes are most indicative of understanding and what kinds of failures are most indicative of a failure to understand? If we applied the same standards to our own behavior, what might we conclude about the relationship between between understanding, knowing and doing?
Gary Lupyan is Professor of Psychology at the University of Wisconsin-Madison. His work has focused on how natural language scaffolds and augments human cognition, and attempts to answer the question of what the human mind would be like without language. He also studies the evolution of language, and the ways that language adapts to the needs of its learners and users.
Hu, J., Mahowald, K., Lupyan, G., Ivanova, A., & Levy, R. (2024). Language models align with human judgments on key grammatical constructions (arXiv:2402.01676). arXiv.
Titus, L. M. (2024). Does ChatGPT have semantic understanding? A problem with the statistics-of-occurrence strategy. Cognitive Systems Research, 83.
Pezzulo, G., Parr, T., Cisek, P., Clark, A., & Friston, K. (2024). Generating meaning: Active inference and the scope and limits of passive AI. Trends in Cognitive Sciences, 28(2), 97–112.
van Dijk, B. M. A., Kouwenhoven, T., Spruit, M. R., & van Duijn, M. J. (2023). Large Language Models: The Need for Nuance in Current Debates and a Pragmatic Perspective on Understanding (arXiv:2310.19671). arXiv.
Aguera y Arcas, B. (2022). Do large language models understand us? Medium.
Lupyan, G. (2013). The difficulties of executing simple algorithms: Why brains make mistakes computers don’t. Cognition, 129(3), 615–636.
_______________________^top^
“Understanding AI”: Semantic Grounding in Large Language Models
Theoretical Philosophy & Center for Behavioral Brain Sciences, U Magdeburg
ISC Summer School on Large Language Models: Science and Stakes, June 3-14, 2024
Fri, June 14, 9am-10:30am EDT
Abstract: Do LLMs understand the meaning of the texts they generate? Do they possess a semantic grounding? And how could we understand whether and what they understand? We have recently witnessed a generative turn in AI, since generative models, including LLMs, are key for self-supervised learning. To assess the question of semantic grounding, I distinguish and discuss five methodological ways. The most promising way is to apply core assumptions of theories of meaning in philosophy of mind and language to LLMs. Grounding proves to be a gradual affair with a three-dimensional distinction between functional, social and causal grounding. LLMs show basic evidence in all three dimensions. A strong argument is that LLMs develop world models. Hence, LLMs are neither stochastic parrots nor semantic zombies, but already understand the language they generate, at least in an elementary sense.
Holger Lyre, Professor of Theoretical Philosophy and member of the Center for Behavioral Brain Sciences (CBBS) at the University of Magdeburg. His research areas comprise philosophy of science, neurophilosophy, philosophy of AI and philosophy of physics. His publications include 4 (co-) authored and 4 (co-) edited books as well as about 100 papers. He has worked on foundations of quantum theory and gauge symmetries and made contributions to structural realism, semantic externalism, extended mind, reductionism, and structural models of the mind. See www.lyre.de
Lyre, H. (2024). “Understanding AI”: Semantic Grounding in Large Language Models. arXiv preprint arXiv:2402.10992.
Lyre, H. (2022). Neurophenomenal structuralism. A philosophical agenda for a structuralist neuroscience of consciousness. Neuroscience of Consciousness, 2022(1), niac012.
Lyre, H. (2020). The state space of artificial intelligence. Minds and Machines, 30(3), 325-347.
_________________________^top^
The Epistemology and Ethics of LLMs
Philosophy, McGill
ISC Summer School on Large Language Models: Science and Stakes, June 3-14, 2024
Thurs, June 6, 9am-10:30am EDT
Abstract: LLMs are impressive. They can extend human cognition in various ways and can be turned into a suite of virtual assistants. Yet, they have the same basic limitations as other deep learning-based systems. Generalizing accurately outside training distributions remains a problem, as their stubborn propensity to confabulate shows. Although LLMs do not take us significantly closer to AGI and, as a consequence, do not by themselves pose an existential risk to humankind, they do raise serious ethical issues related, for instance, to deskilling, disinformation, manipulation and alienation. Extended cognition together with the ethical risks posed by LLMs lend support to ethical concerns in about “genuine human control over AI.”
Jocelyn Maclure professor of political philosophy, McGill University does research on ethics and political philosophy. His book Secularism and Freedom of Conscience (Harvard University Press, 2011), co-authored with Charles Taylor, has appeared in 9 languages. His recent work on artificial intelligence has led him to explore metaphysical questions ranging from the mind-body problem to the enigma of personal identity.
Maclure, J. (2021). AI, explainability and public reason: The argument from the limitations of the human mind. Minds and Machines, 31(3), 421-438.
Cossette-Lefebvre, H., & Maclure, J. (2023). AI’s fairness problem: understanding wrongful discrimination in the context of automated decision-making. AI and Ethics, 3(4), 1255-1269
_________________________^top^
Using Language Models for Linguistics
Department of Linguistics, The University of Texas at Austin
ISC Summer School on Large Language Models: Science and Stakes, June 3-14, 2024
Fri, June 7, 9am-10:30am EDT
Abstract: Today’s large language models generate coherent, grammatical text. This makes it easy, perhaps too easy, to see them as “thinking machines”, capable of performing tasks that require abstract knowledge and reasoning. I will draw a distinction between formal competence (knowledge of linguistic rules and patterns) and functional competence (understanding and using language in the world). Language models have made huge progress in formal linguistic competence, with important implications for linguistic theory. Even though they remain interestingly uneven at functional linguistic tasks, they can distinguish between grammatical and ungrammatical sentences in English, and between possible and impossible languages. As such, language models can be an important tool for linguistic theorizing. In making this argument, I will draw on a study of language models and constructions, specifically the A+Adjective+Numeral+Noun construction (“a beautiful five days in Montreal”). In a series of experiments small language models are treined on human-scale corpora, systematically manipulating the input corpus and pretraining models from scratch. I will discuss implications of these experiments for human language learning.
Kyle Mahowald is an Assistant Professor in the Department of Linguistics at the University of Texas at Austin. His research interests include learning about human language from language models, as well as how information-theoretic accounts of human language can explain observed variation within and across languages. Mahowald has published in computational linguistics (e.g., ACL, EMNLP, NAACL), machine learning (e.g., NeurIPS), and cognitive science (e.g., Trends in Cognitive Science, Cognition) venues. He has won an Outstanding Paper Award at EMNLP, as well as the National Science Foundation’s CAREER award.
K. Mahowald, A. Ivanova, I. Blank, N. Kanwisher, J. Tenenbaum, E. Fedorenko. 2024. Dissociating Language and Thought in Large Language Models: A Cognitive Perspective. Trends in Cognitive Sciences.
K. Misra, K. Mahowald. 2024. Language Models Learn Rare Phenomena From Less Rare Phenomena: The Case of the Missing AANNs. Preprint.
J. Kallini, I. Papadimitriou, R. Futrell, K. Mahowald, C. Potts. 2024. Mission: Impossible Language Models. Preprint.
J. Hu, K. Mahowald, G. Lupyan, A. Ivanova, R. Levy. 2024. Language models align with human judgments on key grammatical constructions. Preprint.
H. Lederman, K. Mahowald. 2024. Are Language Models More Like Libraries or Like Librarians? Bibliotechnism, the Novel Reference Problem, and the Attitudes of LLMs. Preprint.
K. Mahowald. 2023. A Discerning Several Thousand Judgments: GPT-3 Rates the Article Adjective + Numeral + Noun Construction. Proceedings of EACL 2023.
_________________________^top^
AI’s Challenge of Understanding the World
Santa Fe Institute
ISC Summer School on Large Language Models: Science and Stakes, June 3-14, 2024
Thurs, June 6, 3:30pm-5pm EDT
ABSTRACT: I will survey a debate in the artificial intelligence (AI) research community on the extent to which current AI systems can be said to “understand” language and the physical and social situations language encodes. I will describe arguments that have been made for and against such understanding, hypothesize about what humanlike understanding entails, and discuss what methods can be used to fairly evaluate understanding and intelligence in AI systems.
Melanie Mitchell is Professor at the Santa Fe Institute. Her current research focuses on conceptual abstraction and analogy-making in artificial intelligence systems. Melanie is the author or editor of six books and numerous scholarly papers in the fields of artificial intelligence, cognitive science, and complex systems. Her 2009 book Complexity: A Guided Tour (Oxford University Press) won the 2010 Phi Beta Kappa Science Book Award, and her 2019 book Artificial Intelligence: A Guide for Thinking Humans (Farrar, Straus, and Giroux) is a finalist for the 2023 Cosmos Prize for Scientific Writing.
Mitchell, M. (2023). How do we know how smart AI systems are? Science, 381(6654), adj5957.
Mitchell, M., & Krakauer, D. C. (2023). The debate over understanding in AI’s large language models. Proceedings of the National Academy of Sciences, 120(13), e2215907120.
Millhouse, T., Moses, M., & Mitchell, M. (2022). Embodied, Situated, and Grounded Intelligence: Implications for AI. arXiv preprint arXiv:2210.13589.
_________________________^top^
Symbols and Grounding in LLMs
Computer Science, Brown
ISC Summer School on Large Language Models: Science and Stakes, June 3-14, 2024
Fri, June 14, 11am-12:30pm EDT
ABSTRACT: Large language models (LLMs) appear to exhibit human-level abilities on a range of tasks, yet they are notoriously considered to be “black boxes”, and little is known about the internal representations and mechanisms that underlie their behavior. This talk will discuss recent work which seeks to illuminate the processing that takes place under the hood. I will focus in particular on questions related to LLM’s ability to represent abstract, compositional, and content-independent operations of the type assumed to be necessary for advanced cognitive functioning in humans.
Ellie Pavlick is an Assistant Professor of Computer Science at Brown University. She received her PhD from University of Pennsylvania in 2017, where her focus was on paraphrasing and lexical semantics. Ellie’s research is on cognitively-inspired approaches to language acquisition, focusing on grounded language learning and on the emergence of structure (or lack thereof) in neural language models. Ellie leads the language understanding and representation (LUNAR) lab, which collaborates with Brown’s Robotics and Visual Computing labs and with the Department of Cognitive, Linguistic, and Psychological Sciences.
Tenney, Ian, Dipanjan Das, and Ellie Pavlick. “BERT Rediscovers the Classical NLP Pipeline.” Proceedings of the 57th Annual Meeting of the Association for Computational Linguistics. 2019. https://arxiv.org/pdf/1905.05950.pdf
Pavlick, Ellie. “Symbols and grounding in large language models.” Philosophical Transactions of the Royal Society A 381.2251 (2023): 20220041. https://royalsocietypublishing.org/doi/pdf/10.1098/rsta.2022.0041
Lepori, Michael A., Thomas Serre, and Ellie Pavlick. “Break it down: evidence for structural compositionality in neural networks.” arXiv preprint arXiv:2301.10884 (2023). https://arxiv.org/pdf/2301.10884.pdf
Merullo, Jack, Carsten Eickhoff, and Ellie Pavlick. “Language Models Implement Simple Word2Vec-style Vector Arithmetic.” arXiv preprint arXiv:2305.16130 (2023). https://arxiv.org/pdf/2305.16130.pdf
_________________________^top^
What neural networks can teach us about how we learn language
Decision Sciences, HEC Montréal
ISC Summer School on Large Language Models: Science and Stakes, June 3-14, 2024
Tues, June 4, 9am-10:30am EDT
Abstract: How can modern neural networks like large language models be useful to the field of language acquisition, and more broadly cognitive science, if they are not a priori designed to be cognitive models? As developments towards natural language understanding and generation have improved leaps and bounds, with models like GPT-4, the question of how they can inform our understanding of human language acquisition has re-emerged. This talk will try to address how AI models as objects of study can indeed be useful tools for understanding how humans learn language. It will present three approaches for studying human learning behaviour using different types of neural networks and experimental designs, each illustrated through a specific case study. Understanding how humans learn is an important problem for cognitive science and a window into how our minds work. Additionally, human learning is in many ways the most efficient and effective algorithm there is for learning language; understanding how humans learn can help us design better AI models in the future.
Eva Portelance is Assistant Professor in the Department of Decision Sciences at HEC Montréal. Her research intersects AI and Cognitive Science. She is interested in studying how both humans and machines learn to understand language and reason about complex problems.
Portelance, E. & Jasbi, M.. (2023). The roles of neural networks in language acquisition. PsyArXiv:b6978. (Manuscript under review).
Portelance, E., Duan, Y., Frank, M.C., & Lupyan, G. (2023). Predicting age of acquisition for children’s early vocabulary in five languages using language model surprisal. Cognitive Science.
Portelance, E., M. C. Frank, D. Jurafsky, A. Sordoni, R. Laroche. (2021). The Emergence of the Shape Bias Results from Communicative Efficiency. Proceedings of the 25th Conference on Computational Natural Language Learning (CoNLL).
_________________________^top^
Semantic grounding of concepts and meaning in brain-constrained neural networks
Brain, Cognitive and Language Sciences, Freie Universität Berlin
ISC Summer School on Large Language Models: Science and Stakes. June 3-14, 2024
Mon, June 3, 11am-12:30pm EDT
Abstract: Neural networks can be used to increase our understanding of the brain basis of higher cognition, including capacities specific to humans. Simulations with brain-constrained networks give rise to conceptual and semantic representations when objects of similar type are experienced, processed and learnt. This is all based on feature correlations. If neurons are sensitive to semantic features, interlinked assemblies of such neurons can represent concrete concepts. Adding verbal labels to concrete concepts augments the neural assemblies, making them more robust and easier to activate. Abstract concepts cannot be learnt directly from experience, because the different instances to which an abstract concept applies are heterogeneous, making feature correlations small. Using the same verbal symbol, correlated with the instances of abstract concepts, changes this. Verbal symbols act as correlation amplifiers, which are critical for building and learning abstract concepts that are language dependent and specific to humans.
Friedemann Pulvermueller is Professor of Neuroscience of Language and Pragmatics and Head of the Brain Language Laboratory of the Freie Universität Berlin. His main interest is in the neurobiological mechanisms enabling humans to use and understand language. His team accumulated neurophysiological, neuroimaging and neuropsychological evidence for category-specific semantic circuits distributed across a broad range of cortical areas, including modality-specific ones, supporting correlation-based semantic learning and grounding mechanisms. His focus is on active, action-related mechanisms crucial for symbol understanding.
Dobler, F. R., Henningsen-Schomers, M. R. & Pulvermüller, F. (2024). Temporal dynamics of concrete and abstract concept and symbol processing: A brain constrained modelling study. Language Learning, in press.
Pulvermüller, F. (2024). Neurobiological Mechanisms for Language, Symbols and Concepts: Clues From Brain-constrained Deep Neural Networks. Progress in Neurobiology, in press, 102511.
Nguyen, P. T., Henningsen-Schomers, M. R., & Pulvermüller, F. (2024). Causal influence of linguistic learning on perceptual and conceptual processing: A brain-constrained deep neural network study of proper names and category terms. Journal of Neuroscience, 44(9).
Grisoni, L., Boux, I. P., & Pulvermüller, F. (2024). Predictive Brain Activity Shows Congruent Semantic Specificity in Language Comprehension and Production. Journal of Neuroscience, 44(12).
Pulvermüller, F., & Fadiga, L. (2010). Active perception: Sensorimotor circuits as a cortical basis for language. Nature Reviews Neuroscience, 11(5), 351-360.
_________________________^top^
Revisiting the Turing test in the age of large language models
Neuroscience/AI, Princeton University
ISC Summer School on Large Language Models: Science and Stakes, June 3-14, 2024
Thurs, June 13, 9am-10:30am EDT
Abstract: The Turing test was originally formulated as an operational answer to the question, “Can a machine think?”. Turing provided this operational test because there is no precise, scientific definition of what it means to think. But, as can be seen from the large volumes of writing on the subject since his initial essay, Turing’s operational test never fully satisfied all scientists and philosophers. Moreover, in the age of large language models, there is even disagreement as to whether current models can pass Turing’s test, and, if we claim that they cannot, then many humans cannot pass the test either. I will, therefore, argue that it is time to abandon the Turing test and embrace ambiguity around the question of whether AI “thinks”. Much as biologists stopped worrying about the definition of “life” and simply engaged in scientific exploration, so too can neuroscientists, cognitive scientists, and AI researchers stop worrying about the definition of “thought” and simply move on with our explorations of the human brain and the machines we design to mimic its functions.
Blake Richards is Associate Professor in the School of Computer Science and Montreal Neurological Institute at McGill University and a Core Faculty Member at Mila. Richards’ research is at the intersection of neuroscience and AI. His laboratory investigates universal principles of intelligence that apply to both natural and artificial agents. He has received several awards for his work, including the NSERC Arthur B. McDonald Fellowship in 2022, the Canadian Association for Neuroscience Young Investigator Award in 2019, and a CIFAR Canada AI Chair in 2018
Doerig, A., Sommers, R. P., Seeliger, K., Richards, B., Ismael, J., Lindsay, G. W., … & Kietzmann, T. C. (2023). The neuroconnectionist research programme. Nature Reviews Neuroscience, 24(7), 431-450.
Golan, T., Taylor, J., Schütt, H., Peters, B., Sommers, R. P., Seeliger, K., … & Kriegeskorte, N. (2023). Deep neural networks are not a single hypothesis but a language for expressing computational hypotheses. Behavioral and Brain Sciences, 46.
Zador, A., Escola, S., Richards, B., Ölveczky, B., Bengio, Y., Boahen, K., … & Tsao, D. (2023). Catalyzing next-generation artificial intelligence through neuroai. Nature Communications, 14(1), 1597.
Sejnowski, T. J. (2023). Large language models and the reverse turing test. Neural Computation, 35(3), 309-342.
________________________^top^
Emergent Behaviors in Foundational Models
Université de Montréal, MILA
ISC Summer School on Large Language Models: Science and Stakes, June 3-14, 2024
Thurs, June 13, 1:30pm-3pm EDT
Abstract: The field of AI is advancing at unprecedented speed due to the rise of foundation models – large-scale, self-supervised pre-trained models whose impressive capabilities greatly increase with scaling the amount of training data, model size and computational power. Empirical neural scaling laws aim to predict scaling behaviors of foundation models, thus serving as an “investment tool” towards choosing the best-scaling methods with increased compution, likely to stand the test of time and escaping “the bitter lesson”. Predicting AI behaviors at scale, especially “phase transitions” and emergence, is highly important from the perspective of AI Safety and Alignment with human intent. I will present our efforts towards accurate forecasting of AI behaviors using both an open-box approach, when the model’s internal learning dynamics is accessible, and a closed-box approach of inferring neural scaling laws based solely on external observations of AI behavior at scale. I will provide an overview of open-source foundation models our lab has built over the past year thanks to the large INCITE compute grant on Summit and Frontier supercomputers at OLCF, including multiple 9.6B LLMs trained continually, the first Hindi model Hi-NOLIN, the multimodal vision-text model suite Robin, as well as time-series foundation models. I will highlight the continual pre training paradigm that allows train models on potentially infinite datasets, as well as approaches to AI ethics and multimodal alignment. See our CERC-AAI project page for more details: https://www.irina-lab.ai/projects.
Irina Rish is Professor of Computer Science and Operations Research. Université de Montréal and MILA. Her research is on machine learning, neural data analysis and neuroscience-inspired AI, including scaling laws, emergent behaviors and foundation models in AI, continual learning and transfer. https://sites.google.com/view/irinarish/home
Ibrahim, A., Thérien, B., Gupta, K., Richter, M. L., Anthony, Q., Lesort, T., … & Rish, I. (2024). Simple and Scalable Strategies to Continually Pre-train Large Language Models. arXiv preprint arXiv:2403.08763.
Rifat Arefin, M., Zhang, Y., Baratin, A., Locatello, F., Rish, I., Liu, D., & Kawaguchi, K. (2024). Unsupervised Concept Discovery Mitigates Spurious Correlations. arXiv e-prints, arXiv-2402.
Jain, A. K., Lehnert, L., Rish, I., & Berseth, G. (2024). Maximum State Entropy Exploration using Predecessor and Successor Representations. Advances in Neural Information Processing Systems, 36.
__________________^top^
The Global Brain Argument
Philosophy, Florida Atlantic University
Mon, June 3, 3:30pm-5pm EDT
ISC Summer School on Large Language Models: Science and Stakes, June 3-14, 2024
Abstract: Humans, as users of AI services, are like “nodes” in a larger algorithmic system that is a novel form of ‘hybrid’ intelligence. Eventually, closely interconnected parts of the system, fuelled by advancing generative models, global sensor systems, extensive numbers of users and data, might become a “Global Brain” system. My talk will explore the implications of the global brain argument for the extended mind, the nature of knowledge, consciousness, and human flourishing.
Susan Schneider, William F. Dietrich Distinguished Professor of Philosophy of Mind at Florida Atlantic University, is known for her work in the philosophy of cognitive science and artificial intelligence, particularly the nature of consciousness and the potential for conscious AI.
LeDoux J, Birch J, Andrews K, Clayton NS, Daw ND, Frith C, Lau H, Peters MA, Schneider S, Seth A, Suddendorf T. (2023) Consciousness beyond the human case. Current Biology. 2023 Aug 21;33(16):R832-40.
St. Clair, R., Coward, L. A., & Schneider, S. (2023). Leveraging conscious and nonconscious learning for efficient AI. Frontiers in Computational Neuroscience, 17, 1090126.
Schneider, S. (2020). How to Catch an AI Zombie. Ethics of Artificial Intelligence, 439-458.
Schneider, S. (2017). Daniel Dennett on the nature of consciousness. The Blackwell companion to consciousness, 314-326.
________________________^top^
From Word Models to World Models: Natural Language to the Probabilistic Language of Thought
Computational Cognitive Science, MIT
ISC Summer School on Large Language Models: Science and Stakes, June 3-14, 2024
Thurs, June 6, 1:30pm-3pm EDT
ABSTRACT: How do humans make meaning from language? And how can we build machines that think in more human-like ways? “Rational Meaning Construction” combines neural language models with probabilistic models for rational inference. Linguistic meaning is a context-sensitive mapping from natural language into a probabilistic language of thought (PLoT), a general-purpose symbolic substrate for generative world modelling. Thinking can be modelled with probabilistic programs, an expressive representation for commonsense reasoning. Meaning construction can be modelled with large language models (LLMs) that support translation from natural language utterances to code expressions in a probabilistic programming language. LLMs can generate context-sensitive translations that capture linguistic meanings in (1)probabilistic reasoning, (2) logical and relational reasoning, (3) visual and physical reasoning, and (4) social reasoning. Bayesian inference with the generated programs supports coherent and robust commonsense reasoning. Cognitively motivated symbolic modules (physics simulators, graphics engines, and planning algorithms) provide a unified commonsense-thinking interface from language. Language can drive the construction of world models themselves. We hope this work will lead to cognitive models and AI systems combining the insights of classical and modern computational perspectives.
Josh Tenenbaum is Professor of Computational Cognitive Science in the Department of Brain and Cognitive Sciences at MIT, a principal investigator at MIT’s Computer Science and Artificial Intelligence Laboratory (CSAIL), and a thrust leader in the Center for Brains, Minds and Machines (CBMM). His research centers on perception, learning, and common-sense reasoning in humans and machines, with the twin goals of better understanding human intelligence in computational terms and building more human-like intelligence in machines.
Wong, L., Grand, G., Lew, A. K., Goodman, N. D., Mansinghka, V. K., Andreas, J., & Tenenbaum, J. B. (2023). From word models to world models: Translating from natural language to the probabilistic language of thought. arXiv preprint arXiv:2306.12672.
Mahowald, K., Ivanova, A. A., Blank, I. A., Kanwisher, N., Tenenbaum, J. B., & Fedorenko, E. (2023). Dissociating language and thought in large language models: a cognitive perspective. arXiv preprint arXiv:2301.06627.
Ying, L., Zhi-Xuan, T., Wong, L., Mansinghka, V., & Tenenbaum, J. (2024). Grounding Language about Belief in a Bayesian Theory-of-Mind. arXiv preprint arXiv:2402.10416.
Hsu, J., Mao, J., Tenenbaum, J., & Wu, J. (2024). What’s Left? Concept Grounding with Logic-Enhanced Foundation Models. Advances in Neural Information Processing Systems, 36.
_________________________^top^
LLMs, POS, and UG
Psychology, Hunter College, CUNY
ISC Summer School on Large Language Models: Science and Stakes, June 3-14, 2024
Tues, June 4, 1:30pm-3pm EDT
Abstract: Is the goal of language acquisition research to account for children’s behavior (their productions and performance on tests of comprehension) or their knowledge? A first step in either goal is to establish facts about children’s linguistic behavior at the beginning of combinatorial speech. Recent work in our laboratory investigates children’s productions between roughly 15 and 22 months and suggests a combination of structured and less-structured utterances in which structured utterances come to dominate. A rich body of research from our and other laboratories demonstrates knowledge of syntactic categories, structures, and constraints between 22-30 months, along with considerable variability from child to child. Research on “created” languages like Nicaraguan Sign Language suggests language can develop in the absence of structured input. How do children do it; what is the mechanism? What is the best characterization of the input to children? How much variability is there in acquisition? What is the characterization of the end state of acquisition? Are LLMs intended to characterize knowledge? In answering those and other questions, I will distinguish between the notion of poverty of the stimulus and the notion of noisy and incomplete input and present data on children’s early syntactic behavior.
Virginia Valian is Distinguished Professor of psychology at CUNY Hunter College; she is also a member of the linguistics and psychology PhD Programs at the CUNY Graduate Center. Her research on how children learn language supports theories of innate grammatical knowledge – particularly linguistic universals – and investigates the interaction of linguistic knowledge and higher cognitive processing. Her work has potential implications for artificial intelligence, particularly how LLMs are trained, how they generalize from training data, and their ability to represent variation and variability in language acquisition.
Valian, V. (2024). Variability in production is the rule, not the exception, at the onset of multi-word speech. Language Learning and Development, 20(1), 76-78.
Xu, Q., Chodorow, M., & Valian, V. (2023). How infants’ utterances grow: A probabilistic account of early language development. Cognition, 230, 105275.
Pozzan, L. & Valian, V. (2017). Asking questions in child English: Evidence for early abstract representations. Language Acquisition, 24(3), 209-233.
Bencini, G. M., & Valian, V. V. (2008). Abstract sentence representations in 3-year-olds: Evidence from language production and comprehension. Journal of Memory and Language, 59(1), 97-113.
Valian, V. (2013). Determiners: An empirical argument for innateness. In M. Sanz, I. Laka, & M. Tanenhaus. (Eds.). Language down the garden path: The cognitive and biological basis for linguistic structure (Chapter 14, pp 272-279). New York: Oxford University Press.
_________________________^top^
Computational Irreducibility, Minds, and Machine Learning
Wolfram Research, Champaign Illinois
ISC Summer School on Large Language Models: Science and Stakes, June 3-14, 2024
Fri, June 7, 1:30pm-3pm EDT
Abstract: Whether we call it perception, measurement, or analysis, it is how we humans get an impression of the world in our minds. Human language, mathematics and logic are ways to formalize the world. A new and still more powerful one is computation. I’ve long wondered about ‘alien minds’ and what it might be like to see things from their point of view. Now we finally have in AI an accessible form of alien mind. Nobody expected this—not even its creators: ChatGPT has burst onto the scene as an AI capable of writing at a convincingly human level. But how does it really work? What’s going on inside its “AI mind”? After AI’s surprise successes, there’s a somewhat widespread belief that eventually AI will be able to “do everything”, or at least everything we currently do. So what about science? Over the centuries we humans have made incremental progress, gradually building up what’s now essentially the single largest intellectual edifice of our civilization. The success of ChatGPT brings together the latest neural net technology with foundational questions about language and human thought posed by Aristotle more than two thousand years ago.
Stephen Wolfram is a mathematician, computer scientist, physicist, and businessman. He is known for his work in computer science, mathematics, and theoretical physics. A fellow of the American Mathematical Society, he is the founder and CEO of the software company Wolfram Research where he works as chief designer of Mathematica and the Wolfram Alpha answer engine.
Wolfram, S. (2023). What Is ChatGPT Doing … and Why Does It Work? Wolfram Media.
Matzakos, N., Doukakis, S., & Moundridou, M. (2023). Learning mathematics with large language models: A comparative study with computer algebra systems and other tools. International Journal of Emerging Technologies in Learning (iJET), 18(20), 51-71.
Wolfram, S. (2021). After 100 Years, Can We Finally Crack Post’s Problem of Tag? A Story of Computational Irreducibility, and More. arXiv preprint arXiv:2103.06931.
_________________________^top^
Learning, Satisficing, and Decision Making
Linguistics, University of Pennsylvania
ISC Summer School on Large Language Models: Science and Stakes, June 3-14, 2024
Wed, June 5, 9am-10:30am EDT
Abstract: In machine learning, the learner is assumed to be rational, seeking the highest probability, lowest cost, account of the data. This may not be achievable without a tremendous amount of data. Meanwhile, the problem of overfitting remains a formidable challenge: the best hypothesis from one set of data may not generalize well to another. Herbert Simon observed that human learning and decision-making often do not strive for optimal solutions but merely solutions that are good enough (“satisficing”). Language learning provides the most compelling demonstration. Almost all linguistic rules have exceptions but are nevertheless good enough to generalize. A “Tolerance Principle” of learning by satisficing provides a precise and parameter-free measure of what counts as good enough for generalization. In addition to support from empirical linguistic studies, experimental research with infants (e.g., Shi & Emond 2023) suggests that the Tolerance Principle is a domain-general mechanism, and can be applied to social learning and cultural conventionalization, providing a more accurate account of behavioral data than rational decision processes.
Charles Yang is Professor of Linguistics and Computer Science and director of the cognitive science program at University of Pennsylvania. His research concerns language and cognitive development from a computational perspective. The Price of Linguistic Productivity (2016) won the Leonard Bloomfield Award from the LSA.
Martínez, H. J. V., Heuser, A. L., Yang, C., & Kodner, J. (2023). Evaluating Neural Language Models as Cognitive Models of Language Acquisition. arXiv preprint arXiv:2310.20093.
Shi, R., & Emond, E. (2023). The threshold of rule productivity in infants. Frontiers in Psychology, 14, 1251124.
Yang, C. (2016). The price of linguistic productivity. MIT Press.
Yang, C., Crain, S., Berwick, R. C., Chomsky, N., & Bolhuis, J. J. (2017). The growth of language: Universal Grammar, experience, and principles of computation. Neuroscience & Biobehavioral Reviews, 81, 103-119.
_________________________^top^
Towards an AI Mathematician
Mathematics, California Institute of Technology
ISC Summer School on Large Language Models: Science and Stakes ,June 3-14, 2024
Fri, June 7, 11am-12:30pm EDT
Abstract: Mathematics is a hallmark of human intelligence and a long-standing goal of AI. It involves analyzing complex information, identifying patterns, forming conjectures, and performing logical deduction. Many of these capabilities are beyond the reach of current AI, and unlocking them can revolutionize AI applications in scientific discovery, formal verification, and beyond. In this talk, I will present initial steps towards the grand vision of AI mathematicians, taking an approach that combines the generative power of large language models (LLMs) with the logical rigor of formal methods. I will cover our work on using LLMs to (1) prove formal theorems in proof assistants such as Coq and Lean and (2) automatically translate human-written mathematics into formal theorems and proofs—a task called autoformalization. For theorem proving, we introduce the entire system for extracting data, training LLMs to generate proof steps, interacting with proof assistants to search for proofs, and deploying the model to assist human users. For autoformalization, using Euclidean geometry as an example domain, we introduce a neuro-symbolic framework that combines LLMs with SMT solvers and domain knowledge. Finally, we discuss future directions for AI mathematicians beyond theorem proving and autoformalization, including important problems such as automatic conjecturing and applications in natural language and program verification.
Kaiyu Yang is a Computing, Data, and Society Postdoctoral Fellow at Caltech. His research aims to build AI that can understand and reason about mathematics. To that end, he has focused on using machine learning, especially large language models, to prove theorems in formal environments such as Coq and Lean. He has received the Siebel Scholar award. Before joining Caltech, he received a Ph.D. in computer science from Princeton University and bachelor’s degrees in computer science and mathematics from Tsinghua University.
Yang, K., Swope, A., Gu, A., Chalamala, R., Song, P., Yu, S., … & Anandkumar, A. (2024). Leandojo: Theorem proving with retrieval-augmented language models. Advances in Neural Information Processing Systems, 36.
Shulman, M. (2024). Strange new universes: Proof assistants and synthetic foundations. Bulletin of the American Mathematical Society.
Murphy, L., Yang, K et al.(2024, in prep). Autoformalizing Euclidean Geometry. International Conference on Machine Learning (ICML)
Tao, T. (2024, in press). Machine assisted proof. Notices of the American Mathematical Society.
_________________________^top^
Speakers — Abstracts — draft timetable
The Summer School is free for all on Zoom (with live webstreaming for the overflow on YouTube if needed). Registration is also free, and optional, but we recommend registering so you receive notices if there are any programme changes (and so we can know who and how many are attending). Questions and comments can only be aired if you provide your full name and institution. The video recordings for each of the 33 talks and 7 panels are accessible the day after they occur.
ZOOM ACCESS IS FREE.
MEETING NUMBER IS 85718093402
PHYSICAL LOCALE IN MONTREAL: SHERBROOKE PAVILION (SH) LECTURE HALL SH-3620
Past UQÀM COGNITIVE SCIENCE INSTITUTE Summer Schools:
- Categorization 2003: Handbook
- Social Cognition 2008: Program
- Origin of Language 2010: Handbook
- Origin and Function of Consciousness 2012: Program and videos
- Web Science and the Mind 2014: Program and videos
- Reasoning 2016: Program and videos
- The Other Minds Problem: Animal Sentience and Cognition 2018: Program and videos
- Cognitive Challenges of Climate Change 2021: Videos
PROPAEDEUTIC SEMINARS
(September 2023 – April 2024)
videos
Referential Grounding (Vossen)
Transferable Attacks on Aligned Language Models (Fredrikson)
Autotelic Agents that Use and Ground Large Language Models (Oudeyer)
Gesture Semantics: Deictic Reference, Deferred Reference and Iconic Co-Speech Gestures (Lücking)
The Grounding Problem in Language Models is not only about Grounding (Lenci)
What counts as understanding? (Lupyan)
Learning Categories by Creating New Descriptions (Goldstone)
Language Writ Large: LLMs, ChatGPT, Meaning and Understanding (Harnad)
Mechanistic Explanation in Deep Learning (Millière)
Apprentissage continu et contrôle cognitif » (Alexandre)
Falsifying the Integrated Information Theory of Consciousness (Hanson)
LLMs, Patterns, and Understanding (Durt)
LLMs: Indication or Representation? (Søgaard)
Algorithmes de Deep Learning flous causaux » (Faghihi)
Robotic Grounding and LLMs: Advancements and Challenges (Kennington)
Enactivist Symbol Grounding: From Attentional Anchors to Mathematical Discourse (Abrahamson)
The Debate Over “Understanding” in AI’s Large Language Models (Mitchell)
Rethinking the Physical Symbol Systems Hypothesis (Rosenbloom)
Symbols and Grounding in LLMs (Pavlick)
From the History of Philosophy to AI: Does Thinking Require Sensing? (Chalmers)
Grounding in Large Language Models: Functional Ontologies for AI (Mollo)
LLMs are impressive but we still need grounding to explain human cognition (Bergen)
Conscious processing, inductive biases and generalization in deep learning (Bengio)
Active inference and artificial curiosity (Friston)
Cognitive architectures and their applications (Lebière)
Constraining networks biologically to explain grounding (Pulvermüller)
Hello, when will the recordings be made available? I am from a different time zone and had to miss most of the sessions. Really hope to catch up on this amazing series. Thank you very much!
LikeLike
They should start appearing on YouTube in 1-2 days.
LikeLike